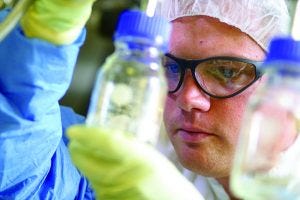
Process sampling at Sanofi Genzyme
(www.sanofi.com)
As head of bioanalytics at Sanofi, Claire Davies leads a team of >90 people involved in development, qualification, and transfer of methods to internal and external commercial quality control units; characterization, comparability, and developability of proteins and gene therapies; and analytical support for up- and downstream development, production, product release, and stability testing. She has served in a number of roles over 18 years with Sanofi, from chemistry, manufacturing, and controls (CMC) leadership of preclinical to commercial products (leading and coordinating multidepartmental teams and mentoring analytical subteam leaders) to writing regulatory submissions and participating in regulatory inspections. She holds a bachelor of science in biochemistry from the University of Surrey in the United Kingdom and a PhD from the University of London, and her postdoctoral work was at St. Bart’s and the Royal London Medical College and Harvard Medical School. For this featured report, we spoke about the importance of teams and technology in rapid analytical development and the increasing role that “big data” has to play in quality by design (QbD).
Single-Use Technologies
Do you see disposables making inroads in analytical laboratories as much as they are on the process side? If so, is there a way they can improve efficiencies? Or have they just slowed things down by adding another kind of testing that must be done? Disposable laboratory supplies are frequently used in analytics, from tubes and flasks to preassembled cartridges. Expanding the use of disposable measuring devices could be feasible depending on the complexity of the analysis and its cost effectiveness. If the analysis is relatively simple, and the devices can be manufactured in an inexpensive and robust way, then it has potential. For example, single-use biosensors and probes would be beneficial for in-line analysis, which would assist in their integration into the bioprocesses. For complex systems requiring precision measurement and control, however, the calibration/qualification burden would outweigh the benefits of disposability. The types of plastics used would need to be selected carefully to prevent extractable and leachable concerns (e.g., interfering peaks or false positives). Other areas where single-use technologies might be beneficial include cell- and molecular-based assays, for which segregation is required to prevent crosscontamination.
Automation and High-Throughput Screening
We’ve seen some impressive shortening of cell-line development through automation. How are robotics and other such technologies changing the game in analytical laboratories? Integrating automation and robotics into analytical laboratories is key to accelerating assay turnaround and throughput. With increased implementation of QbD and multivariate analysis in process and formulation development, the number of samples to handle has increased dramatically. Automation also has proved useful in streamlining developability and stress studies, from set-up to sample analysis. Even with good manufacturing practice (GMP) release and stability testing, automation has improved the precision of cell-based end-point assays, activity assays, and infectious titer assays. I believe the next stage in this evolution will be to integrate sample preparation and analysis more seamlessly using robotic arms to connect multiple instruments to enable fully automated workflows. Key to successful implementation of this technology is a diverse team of automation scientists, development scientists, and advanced data scientists working closely together.
Similarly, we’ve seen high-throughput approaches for screening media formulations and chromatographic conditions. What can high-throughput screening (HTS) do for early product characterization and/or assay development? HTS can be very beneficial in developability and early product characterization to understand a molecule’s susceptibility to different stresses. Using that information, we can select the best development candidates; study changes in their quality attributes with respect to potency, thereby identifying the critical quality attributes (CQAs); and streamline the choice of stability-indicating assays to include. HTS also can be beneficial in method development to confirm that a molecule fits the platform design space for key analytical quality attributes — for example, time and temperature in a purity capillary gel electrophoresis (CGE) method. For more product-specific assays or new technology development, HTS can be used to identify key analytical attributes, which then can be fine-tuned quickly to accelerate method development.
Process Analytical Technology
In-process testing traditionally has been a major task for analytical laboratories. Is on-the-floor/in-process (at/on-line) testing making a difference yet? Does this give analysts more time to work on characterization and other activities? The use of process analytical technologies (PAT) for biologics remains in its infancy, so we have yet to realize its true potential. However, the potential of feedback control is very exciting with development of continuous manufacturing processes and real-time data analytics from biosensors, Raman spectroscopy, and Fourier-transform infrared (FTIR) spectroscopy. Successful implementation of some at-line and in-line analytics have been reported in the process development space: e.g., multimodal chromatography (MMC), multiple-attribute monitoring (MAM), and inline analysis of cell metabolites and concentration. Before the investigational new drug (IND) application stage, the benefits of PAT are limited to more direct analytics such as the MAM and MMC because development of chemometric models takes time and requires significant amounts of off-line data. Hence, the benefits are more likely to be realized during late-stage development and through product life-cycle management.
Platforms and Prior Knowledge
Even as new methods and approaches are changing things, prior-knowledge assessments remain important. What kinds of analytical platform approaches can help to speed things along? Well-defined and robust analytical platforms are key to accelerating and streamlining all stages of product development. If an analytical platform is well-developed with an understanding of a method’s performance and robustness for multiple products of similar modality, then prior knowledge can be used to limit the extent of method development and qualification for new candidates. This is extremely beneficial before the IND stage because it enables method development to be limited to key analytical attributes that are known to be molecule-specific, thus enabling rapid method qualification. If method development and qualification activities can be defined sufficiently, then associated reports can be templated to improve report writing and reviewing timelines. Even in late-stage development, significant savings can be realized by decreasing the extent of robustness experiments and limiting the number of analytical methods to be maintained.
Using platforms across multiple groups and sites can streamline assay transfer. Combined with use of common assay controls, they can ensure platform performance, improving the efficiency of root-cause investigations for laboratory error events (e.g., vendor-sourced reagent lot variability). If crossfunctional teams also use the same platform methods, then consistent data can be collected across a product’s life cycle throughout developability, cell-line selection, formulation, process development, and manufacturing. That allows data to be leveraged across groups for improved process and product understanding with limited overlap.
However, effective platform management is required for those benefits to be leveraged fully. Making too many changes to a platform requires exhaustive change control and causes knowledge loss in transfers; changing too little makes a platform obsolete. Defining a life-cycle management process for a platform and sticking to it are key to successful platform maintenance.
Crossfunctional Teams
Can it help to include process experts in analytical decision-making — and vice versa? What other functions can contribute to analytical efficiencies? Absolutely, it is imperative to work closely with process and formulation development teams to define an analytical control strategy and develop a target profile for method development. If those tasks are completed “in a vacuum,” quality attributes might not be assessed for their criticality, and methods designed might not be suitable for their intended purpose. With an in-depth understanding of analytical tools, CQAs, and structure–function information, analytical scientists are key contributors to process decisions — providing a perspective on the potential effects of process changes on product quality. Our knowledge of orthogonal tools and CQAs also can be used to streamline process optimization experiments by limiting unnecessary analysis and defining efficient sample-transfer approaches to limit analysis steps.
Even before process development starts, analytical scientists are collaborating with cell-line development teams to provide product-quality information on the best clones and constructs and working with researchers to transfer knowledge on potency and mechanism of action (MoA) for establishment of suitable assays. Front-loading those items in the development life cycle improves early product understanding and speed to IND. For example, even though binding assays are used routinely to release early clinical lots, it is important to initiate the development of a cell-based assay early. Even in characterization mode, it will provide key information on how different materials might behave in a clinical setting, and it facilitates the study of structure–function relationships.
It’s important that analytical, nonclinical, and clinical teams form a tight partnership working together to define CQAs and justify specifications using prior and current knowledge. Strong collaborations with regulatory and clinical supply groups facilitate period-of-use extensions and opportunities to understand the agencies’ position on alternative approaches. All in all, analytical groups are the “sensory organs” of product development, providing data and information so that the best product can be brought rapidly into clinical testing.
Outsourcing
What aspects of working with a contract development and manufacturing organization (CDMO) can slow things down in product characterization — and how can sponsors mitigate those problems? Are contract testing laboratories a time-saver? Outsourcing can be problematical with challenging method transfers, high invalid-assay rates, and extensive investigations leading to inevitable delays. Although it takes time and patience, finding a good outsourcing partner with the appropriate skills and then working with that company to develop a good relationship is key. If you are outsourcing complex methods and processes because you don’t have the skills internally, then finding a skilled partner is imperative. If you are outsourcing to extend your resources and handle capacity peaks, then working to transfer routine methods for common modalities appears to work best. It’s also beneficial to use an outsourcing partner that will establish your platform methods in house. With methods coqualified at both sites, early batches can be tested at either site, thereby mitigating delays from technical issues or conflicting priorities.
Some of the biggest challenges with outsourcing are maintaining effective communication and delivering on time with appropriate quality. It is therefore important to define timelines and deliverables clearly in the contract, with enough detail to set the stage for success. For example, if a contact laboratory is asked to develop a method for a project, then providing an analytical target profile will ensure that expectations regarding the performance and ranges expected of that method are established up front. That way, it will be clear whether the deliverable has been achieved successfully. If technical or project-management issues arise, then a sponsor should consider sending a “person in lab” or team to work alongside the outsourcing team. That can help prevent frustration building up with either party so that a successful resolution can be found quickly.
As to whether using contract testing laboratories saves time, it depends on why, who, what, and how.
Quality By Design
Many companies wait to implement QbD later in product development — e.g., during clinical testing, when GMPs must apply. But can it help to include risk assessment and identifying quality attributes in early development — or does that inevitably slow things down? Before the IND stage, it can be challenging to define a quality target product profile (QTPP) and complete critical quality attribute (CQA) assessments. The clinical plan is still in development, and the process and formulation are being optimized. So the strength, process impurities, and excipient lists can be in flux, making it challenging to document them within rapid timelines. Even if such assessments cannot be completed formally, however, generating a draft document or outline using QbD principles is important to ensure that all functions represented in the CMC team are aligned for the drug candidate to be developed. A QTTP outline and potential CQA list are important to guide process teams, define the analytical control strategy, and provide information on drug-product dosage and configuration.
With common modalities, some aspects can be accelerated using prior knowledge. For example, some quality attributes can be designated as noncritical quality attributes and others as obligatory CQAs, thereby reducing the quality attribute list. The use of templates and databases containing key literature with nonclinical and clinical data also can streamline scoring and generate a first draft early in development.
Predictive Modeling
Related to the above, how can in-silico and in-vitro models help improve early product understanding and assay development? Opportunities to use in silico analysis to facilitate assay development are being reported more than ever before: from in silico models to predict separation behavior of molecules in high-performance liquid chromatography (HPLC) to computer-based simulation programs for designing polymerase chain reaction (PCR) probes and primers. Using such models is feasible to accelerate method development, and they show promise in targeting initial conditions. Combining in silico models with other information can be powerful for identifying targets for development. In silico data can be used to identify off-target effects of cell therapies, for example, which can be validated with biochemical and cell-based data. The targets then can be monitored using next-generation sequencing (NGS) for product characterization (1).
In early product understanding, in silico models can be used to study solution behavior and its impact on biophysical properties, then predict a molecule’s susceptibility to stress and potential to experience post-translational modifications such as deamidation. With host-cell proteins (HCPs), in silico and in vitro data can be used to study immunogenicity risk of different HCPs, thereby informing project teams of the potential risks that need to be studied, controlled, or eliminated. Predictive modeling is an exciting field providing us with approaches to facilitate product understanding. However, it is important to validate the data to confirm each model.
Caveats
When quality and safety are at stake, it can be a mistake to focus only on speed. How might you caution others when it comes to shortening analytical timelines? Reducing analytical timelines is all about working together with the CMC team to take calculated risks and using prior knowledge to make informed decisions. One of the common pitfalls to avoid is assuming “one size fits all.” For example, if we assume that a molecule fits a platform and that the platform data are relevant without verifying that through appropriate assessments, then we can wind up with an assay that fails method qualification, leads to invalid tests, or delays lot release. Potency and strength assays present different challenges, but rushing them can lead to poor performance and the inability to differentiate clinical doses.
A second challenge is to define a representative batch in the final formulation while many activities are occurring in parallel. It is tempting to use an earlier batch to save time, but that would need careful consideration regarding potential impacts on method development and qualification. Finally, pushing hard to reduce timelines often leads to higher deviation rates and lengthy investigations that cost more time than it saves. Hence, thoughtful consideration of how we simplify, digitalize, and improve processes is key to making it across the finish line on time.
Data, Data, Data
With increased assay throughput, MAMs, PAT, and product understanding studies, the amount of information an analytical scientist generates and must interpret has skyrocketed. Traditional data-storage and analytical approaches are inadequate to the task. Building an appropriate information technology (IT) infrastructure and providing related support will allow companies to help analytical scientists effectively leverage prior knowledge to justify specifications, build platforms, define CQAs, and study structure–function relationships. Superior access to data and appropriate data-visualization tools will allow information to be shared seamlessly throughout crossfunctional groups, facilitating data-sharing efficiencies, providing for improved process understanding, and making lot-release acceleration possible. Digital transformation is a key enabler to improve speed to IND (2–7).
References
1 O’Connell DJ, et al. In Silico, Biochemical, and Cell-Based Integrative Genomics Identifies Precise CRISPR/Cas9 Targets for Human Therapeutics. European Society of Gene and Cell Therapy 27th Annual Meeting: Barcelona, Spain, October 2019.
2 Richelle A, von Stosch M. From Big Data to Precise Understanding: The Quest for Meaningful Information. BioProcess Int. February 2020; https://bioprocessintl.com/manufacturing/information-technology/systems-biology-tools-for-big-data-in-the-biopharmaceutical-industry.
3 Warschat J, Bergemann R. Using Blockchain Technology to Ensure Data Integrity: Applying Hyperledger Fabric to Biomanufacturing. BioProcess Int. March 2020; https://bioprocessintl.com/manufacturing/information-technology/using-blockchain-technology-to-ensure-data-integrity-applying-hyperledger-fabric-to-biomanufacturing.
4 Rios M, Roesch M. Smart Sensors and Data Management Solutions for Modern Facilities. BioProcess Int. October 2019; https://bioprocessintl.com/analytical/pat/smart-sensors-and-data-management-solutions-for-modern-facilities.
5 Abel J. Using Data and Advanced Analytics to Improve Chromatography and Batch Comparisons. BioProcess Int. October 2018; https://bioprocessintl.com/downstream-processing/chromatography/using-data-and-advanced-analytics-to-improve-chromatography-and-batch-comparisons.
6 Montgomery SA, Graham LJ. Big Biotech Data: Implementing Large-Scale Data Processing and Analysis for Bioprocessing. BioProcess Int. September 2018; https://bioprocessintl.com/manufacturing/information-technology/big-biotech-data-implementing-large-scale-data-processing-and-analysis-for-bioprocessing.
7 Whitford W. The Era of Digital Biomanufacturing. BioProcess Int. March 2017; https://bioprocessintl.com/manufacturing/information-technology/the-era-of-digital-biomanufacturing.
Claire Davies, PhD, is associate vice president of bioanalytics at Sanofi Genzyme, 1&5 The Mountain Road, Framingham, MA 01701; [email protected], https://www.sanofigenzyme.com. The views and opinions expressed in this article are those of the author and do not necessarily reflect the official policy or position of any other agency, organization, employer, or company.