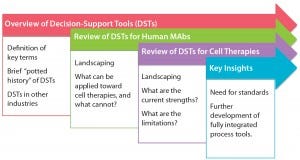
Figure 1: Summary of discussion
Industrial-scale manufacturers in a number of fields — from automobiles to biotherapeutics — have long relied on powerful computational and mathematical tools to aid in the scale-up, optimization, quality control, and monitoring of product development (1–5). Typical process pathways are highly multifactorial, with numerous branch points, feedback steps, instrumental attributes, and target parameters. Moreover, margins for error are minimal for most industrial processes, requiring high standards of precision from industrial and operational pathways (6). For those reasons, the complexity of process engineering and process pathway design necessitates that modeling and decision-support tools (DSTs) be used to ensure high-quality and economically viable end products.
Biologics are no exception to this trend of growing DST use in industrial processes. Compared with classical (small-molecule) pharmaceuticals, biopharmaceuticals exhibit more complex, more expensive, and more delicate production pathways, with long development times and stringent quality standards (7–9). In addition to concerns regarding adverse patient outcomes (which drive pharmaceutical regulation), biologics also must contend with risks of host compatibility, pathogen-carrying tissues, and relatively short product shelf lives, to name just a few issues (10, 11). To contend with those multifaceted challenges, biopharmaceutical researchers and manufacturer working in tandem have developed a suite of decision-support tools (DST) for optimizing the development of products such as monoclonal antibody (MAb) therapeutics (12). Varying in scope and construction, these tools already have exhibited a remarkable propensity to reduce costs, risks, and product complications while increasing end-stage quality (9, 13–15).
However, that is far from true for all biologics. Although optimization, risk modeling, process design, and decision-support systems have been exploited successfully for protein-based biopharmaceuticals, use of such methods remains limited for cell therapy engineering pathways. That is in part because of a fundamental lack of understanding about the key critical unit operations, product critical quality attributes (CQAs), and process analytical technologies (PATs) necessary to drive clinical-stage quality improvements in cell biomanufacturing (16, 17). Notably, these methods have gained significance as cells become more vital diagnostic and therapeutic tools in biomedical engineering, and enabling scalability of such technologies has become critical (18).
Here, we address that unmet need for cell therapy biomanufacturing, in part by leveraging existing decision-engineering frameworks for biopharmaceuticals to build on the nascent body of published work geared towards cell therapies. With a focus on allogeneic human stem cells for human therapeutic use — the largest and most “scale-ready” therapeutic class of cells — below we review, analyze, and synthesize decision-support and optimization tools for large-scale cell therapy bioprocessing with a goal of achieving the following objectives:
To define the fundamentals of decision-support and optimization tools, provide a basic history of developments in the field, and unearth examples from other industries, fields, or sectors of successful developments that might be applied toward cell therapy bioprocessing
To characterize the current state of the art in modeling, design, and optimization tools for human allogeneic cell therapy and MAb bioprocessing, alongside a basic overview of the bioprocessing technologies modeled by these tools
To identify key trends, drivers of convergence and divergence, and remaining gaps in DSTs for human cell therapies.
Overview of Decision-Support Tools
DSTs are software instruments that allow for structured decision-making through the use of models and analytical techniques (19, 20). In general, these systems promise to increase operational efficiency, aid in problem-solving, streamline organizational structure and management processes, and facilitate intergroup coordination (21, 22). Such tools first emerged during the late 20th century as part of the inception and explosion of an information and communications technology industry as catalyzed by the growth in computational power dictated by Moore’s Law (23). They were initially implemented in the airline industry, which intrinsically faces highly complex processes in both ground and air operations (24, 25). Decision-support simulations quickly presented a means of reducing travel delays through operational process optimization. Subsequent successes have been replicated in a number of industries, including manufacturing, restaurant services, and banking (23). Decision-support systems do not obviate the need for human control and decision-making; they merely assist to that end.
With the advent of such paradigm shifts in computing as cloud storage, big data, and machine learning, DSTs have grown in power and relevance to industry, rendering such software critical to competitive businesses of all types (21). But in practice, decision-support programs can take on a number of forms based on a wide range of frameworks. These tools can be stochastic or deterministic in nature, varying in their level of automation, application, commercialization, and complexity. When coupled with advanced visualization tools, decision-support software can become particularly powerful through a broadened range of end-user accessibility (19).
Landscaping MAb and Cell Therapy DSTs
We reviewed existing DSTs designed specifically for biotherapeutic process engineering, optimization, and development by searching PubMed, patent databases (for PATs), and trade literature. Although a number of software packages and process suites have been developed for biological applications, they heretofore have been left largely unassessed for broader trends, traits of convergence and divergence, degree of application, and existing gaps.
To inform further DST development for cell therapies, we examined existing process systems along a number of dimensions: e.g., cell type, process scale, modeling techniques used, and degree of validation. Initially, we conducted this landscaping analysis for MAbs because of the wealth of modeling tools designed for that therapeutic class as well as its broad representation of biologic medicinal products in general. We followed that with a review of cell therapies specifically, for which the array of results was far narrower but more tangibly relevant to the questions at hand. Table 1 summarizes our results.
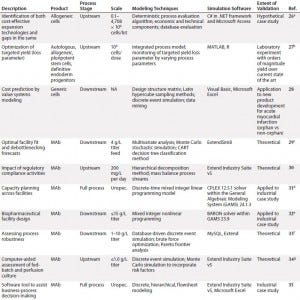
Table 1a: Summarized landscaping results of decision support tools (DSTs) for monoclonal antibodies (MAbs) and cell therapies (continued)
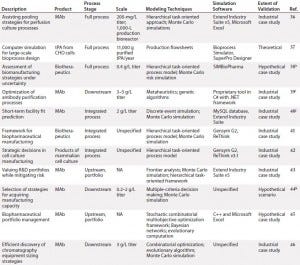
Table 1b: (continued) Summarized landscaping results of decision support tools (DSTs) for monoclonal antibodies (MAbs) and cell therapies
Monoclonal Antibodies: Reviewing the available set of DSTs published for biopharmaceuticals — specifically, MAbs — yielded a deeper understanding of the computational body of work that has enabled economical scale-up of these medical products by manufacturers over a comparatively short period. One immediate trend became apparent: Despite their common application, such models are nonetheless highly heterogeneous. Indeed, not only do they span different subsets of the bioprocessing pathway (thus positing distinct inputs and outputs), they typically use different methods, frameworks, software tools, scales, and validation methods and lie at different levels of maturity.
In spite of that diversity, a few themes have emerged. For example, although a few models took into account inherent risks — and related stochasticities — strewn throughout the manufacturing process, the DSTs were by and large deterministic. That leaves a need for more risk-flexible tools yet unmet. And few available models construct a mass balance of process streams, which makes it more difficult for users to tie simulation steps to real-world bioprocessing decision trees. Also, the overwhelming majority of available DSTs are highly theoretical in scope, with validation only to theoretical constructs or — in a few instances — industrial case studies.
Cell Therapy: Compared with the broad array of DSTs for MAbs, the availability of published tools for cell therapies is substantially limited. In fact, we identified only three such models for cell therapies, which limited the degree to which trends could be meaningfully extracted. Still, it is clear from this landscaping that just as with MAbs, cell therapy DSTs are highly heterogeneous. Each possesses a distinct structure, niche, and approach, and they vary in the degree of “shovel-readiness” for a given biomanufacturer.
Additionally, note that none of those existing tools adopted a clinical lens in examining the simulated cell therapy products, which leaves unaddressed a critical goal of biomanufacturing management: to tie process to clinical products. Above all, though, the landscaping here showcased a substantial need for an assortment of options with increased depth and breadth to catalyze the scaling-up of cell therapy production in coming years.
Moving Forward
Repositioning MAb DSTs for Cell Therapy: Many DST applications explored for MAbs have not yet been explored for cell therapy. Particularly for allogeneic therapies, which conform more closely to “traditional” biomanufacturing paradigms, some existing DSTs could be adapted to explore the many as-yet untapped niches of the manufacturing process.
Autologous cell therapies require a fundamentally different manufacturing approach, and we did not identify any DSTs designed specifically for such processes. Given that there are many perceived challenges for autologous therapy manufacturing (47), development of appropriate DSTs to keep the costs of developing such processes low could be a significant advantage. Furthermore, later-stage DSTs (such as those focusing on regulation) or higher level strategic tools (such as those focusing on portfolio management) might be readily applied to both autologous and allogeneic cell therapies.
Missing Pieces — Intellectual Property and Regulation: A particularly interesting finding is that the intellectual property (IP) surrounding bioprocesses has thus far been largely neglected by DSTs. Traditionally, the key IP surrounding small-molecule drugs has protected each molecular entity itself as well as its therapeutic application (Figure 2). However, practices began to change with the development of biologics. Multiple patents could cover a single therapeutic entity, and complex processes involved in modifying and developing each entity could be patented to prolong its exclusivity period. For example, the commercially critical patents for anti–tumornecrosis-factor (anti-TNF) methotrexate define the difference between a low-cost and established small molecule and a higher cost MAb (48, 49).
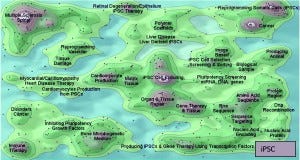
Figure 2: Comparing the patent landscapes for induced pluripotent stem cell (top) and a small-molecule drug candidate (MK-1775, bottom) highlights need for intellectual property consideration in cell therapy DST — search and analysis conducted in Thomson Innovation and Thomson Data Analyzer (TDA) software from Thomson Reuters; themescape maps produced using TDA. Both landscapes reveal patents in many therapeutic areas. However, a key difference is that many iPSC patents cover processing (reprogramming, production, culture, selection, screening, and scaffolds). They could lead to restrictions surrounding core processing technologies, potentially limiting the applicability of those otherwise identified by a DST as optimal. We have observed similar trends in as-yet unpublished analyses of other regenerative medicine areas.
However, even in portfolio analysis of DSTs for biopharmaceuticals, we found that intellectual property was not included as a variable that could affect decision making. That is despite the fact that it is critically important to the industry and is directly applicable to processing (50, 51).
The case for including IP in cell therapy DST is even more compelling. For regenerative medicines such as cell therapies, process patents may be fundamentally important to both research and transitions to commercialization (Figure 2). For example, a review of the induced pluripotent stem cell (iPSC) landscape reveals that the distribution of patents around core technologies for generation and differentiation could lead to appropriate conditions for patent “thicket” formation (52). Because the aim of DSTs often is to identify an optimal process, IP must be taken into account. If it is not, complexities and costs of licensing technologies, for example, could become prohibitively expensive for a process that would otherwise maximize cost efficiency and output.
The regulatory landscape is considered in a few DSTs, but generally it is another overlooked yet critically important consideration. Process models often identify the least expensive technologies without assessing the feasibility of such processes from a regulatory perspective. For cell therapies and other regenerative medicines, meeting regulatory requirements often is considered a challenge. Indeed, the very nature of living products makes demonstrating comparability, consistency, and quality difficult (53, 54). A common adage in the cell therapy industry claims that “the product is the process,” which rightly implies that changing a process can and often does affect the product. Assessing how such changes could influence regulatory compliance therefore would be valuable.
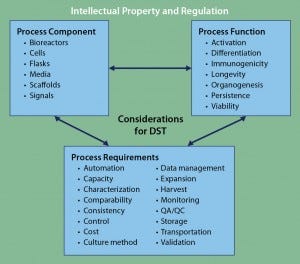
Figure 3: Framework for DST approaches to cell therapy; literature review and analysis of existing tools revealed three core areas for DST consideration: process components, process functions, and process requirements. Despite considerable cross-talk among those areas, overarching considerations of intellectual property and regulation can be applied to complement any DST. At present, no such DST exists for cell therapies.
Of course, it would be impractical for all DSTs to incorporate regulatory- and IP-based decision aspects. However, for practical users of such tools, they are both additional complications of which to be aware. DST development focusing specifically on IP and/or regulation — particularly for cell therapies — would strongly complement and supplement other existing approaches. Such a program could become an important overarching tool in the existing DST ecosystem (Figure 3).
End-to-End DST: The models we assessed exhibited a substantial degree of heterogeneity, with varying software environments, programming languages, syntax, and frameworks making them incapable of “talking” to each other in any meaningful way. With many of these models possessing complementary strengths and capabilities, that mutual incompatibility presents a significant lost opportunity for the biomanufacturing industry. Development of standards such as those published by the International Organization for Standardization (ISO) for bioprocess modeling tools could pave the way for piecing these diverse models together into a more comprehensive “end-to-end” suite of systems.
Furthermore, whereas current process tools model process-chain elements with substantial depth, end- or regulatory-stage attributes (e.g., for quality assurance and control and clinical operations) often are excluded. The lack of distinction between process and product for cell therapy biomanufacturing necessitates that clinical-stage use be closely considered in every cell therapy process design. To truly enable scale-up of such therapies, decision models need to be fully integrated: from inputs to clinic and back.
Room for Expansion
In general, our landscaping analysis has revealed a broad platform of decision-support systems developed both academically and industrially for biologics. They are imperfect, but these tools span a broad range of applications. Given the success of MAbs, such tools have been clearly sufficient to meet many of their demands in the burgeoning biomanufacturing industry. But for cell therapies specifically, ample room remains for growth. The current generation of DST developers can learn and reposition technologies from the biopharmaceutical DST portfolio while also prospectively addressing the idiosyncratic issues of the cell therapy industry (e.g., IP and regulatory compliance). We hope that the growth and success of a profitable and effective cell therapy industry thus will be facilitated.
Acknowledgments
We express sincere thanks to the following organizations that have contributed to the CASMI Translational Stem Cell Consortium (CTSCC) as funding and events partners, without whom the consortium and the benefits it will bring to stem cell translation would be constrained: GE Healthcare, the Center for Commercialization of Regenerative Medicine (CCRM), Sartorius Stedim Biotech (formerly TAP Biosystems), Lonza, the California Institute for Regenerative Medicine (CIRM), the Strategies for Engineered Negligible Senescence (SENS) Research Foundation, UK Cell Therapy Catapult, NIH Centre for Regenerative Medicine, the New York Stem Cell Foundation (NYSCF), ThermoFisher Scientific, Eisai, Medipost (US), Medipost (Korea), Celgene, Roche and Oxford Biomedica. Author Brindley gratefully acknowledges personal funding from the Oxford Musculoskeletal National Institute for Health Research (NIHR), the Saïd Foundation, and the SENS Research Foundation. Author Smith gratefully acknowledges support from the CASMI Translational Stem Cell Consortium (CTSCC). And author Arshad acknowledges support from a SENS Research Foundation Summer Scholar Award.
Conflict of Interest Statement
This article represents the authors’ individual opinions and may not necessarily represent the viewpoints of their employers. Brindley is a stockholder in Translation Ventures Ltd. (Charlbury, Oxfordshire, UK) and IP Asset Ventures Ltd. (Oxford, Oxfordshire, UK), companies that among other services provide cell therapy biomanufacturing, regulatory, and financial advice to pharmaceutical clients. Smith is a consultant with IP Asset Ventures Ltd. Brindley also is subject to the CFA Institute’s codes, standards, and guidelines, so he must stress that this piece is provided for academic interest only and must not be construed in any way as an investment recommendation. Additionally, at time of publication, Brindley and the organizations with which he is affiliated may or may not have agreed and/or pending funding commitments from the organizations named herein.
References
1 Klabjan D. Large-Scale Models in the Airline Industry: Column Generation. Desaulniers G, Desrosiers J, Solomon M, Eds. Springer: Berlin–Heidelberg, Germany, 2005; 163–195.
2 Kodiyalam S, et al. Multidisciplinary Design Optimization of a Vehicle System in a Scalable, High Performance Computing Environment. Struct. Multidiscip. Optim. 26(3– 4) 2004: 256–263.
3 Liao X, et al. Multiobjective Optimization for Crash Safety Design of Vehicles Using Stepwise Regression Model. Struct. Multidiscip. Optim. 35(6) 2008: 561–569.
4 Schmidt FR. Optimization and ScaleUp of Industrial Fermentation Processes. Appl. Microbiol. Biotechnol. 68(4) 2005: 425–435.
5 Wang X, et al. Scale-Up and Optimization of Biohydrogen Production Reactor from Laboratory-Scale to IndustrialScale on the Basis of Computational Fluid Dynamics Simulation. Int. J. Hydrog. Energy. 35(20) 2010: 10960–10966.
6 Bulkeley WM. Innovation Depends on a Robust Manufacturing Sector. MIT Technol. Rev. 7 July 2011; www.technologyreview.com/news/424598/innovation-depends-on-a-robustmanufacturing-sector.
7 DiMasi JA, Grabowski HG. The Cost of Biopharmaceutical R&D: Is Biotech Different? Manag. Decis. Econ. 28(4–5) 2007: 469–479.
8 Rathore AS, Winkle H. Quality By Design for Biopharmaceuticals. Nat. Biotechnol. 27(1) 2009: 26–34.
9 Molowa DT, Mazanet R. The State of Biopharmaceutical Manufacturing. Biotechnol. Ann. Rev. 9, 2003: 285–302.
10 Lee SJ, Williams LA. Patient-Reported Outcomes for Acute Graft-Versus-Host Disease Prevention and Treatment Trials. Biol. Blood Marrow Transplant. 16(3) 2010: 295–300.
11 Ratcliffe E, Thomas RJ, Williams DJ. Current Understanding and Challenges in Bioprocessing of Stem Cell-Based Therapies for Regenerative Medicine. Br. Med. Bull. 100, 2011: 137–155.
12 Lakhdar K, et al. Medium Term Planning of Biopharmaceutical Manufacture Using Mathematical Programming. Biotechnol. Progr. 21(5) 2005: 1478–1489.
13 Farid SS, Washbrook J, TitchenerHooker NJ. Modelling Biopharmaceutical Manufacture: Design and Implementation of SimBiopharma. Comput. Chem. Eng. 31(9) 2007: 1141–1158.
14 Chhatre S, et al. A Decision-Support Model for Evaluating Changes in Biopharmaceutical Manufacturing Processes. Bioprocess Biosyst. Eng. 30(1) 2007: 1–11.
15 Shen J, et al. Decision Support Systems in Drug Discovery. Min. Rev. Med. Chem. 4(10) 2004: 1019–1028.
16 Davie NL, et al. Streamlining Cell Therapy Manufacture. BioProcess Int. 10(3) 2012: S24–S28, S49.
17 Brindley DA, Wall IB, Bure KE. Automation of Cell Therapy Biomanufacturing. BioProcess Int. 11(3) 2013: S18–S25.
18 Mason C, et al. Cell Therapy Industry: Billion Dollar Global Business with Unlimited Potential. Regen. Med. Fut. Med. 6(3) 2011: 265–272.
19 Shim JP, et al. Past, Present, and Future of Decision Support Technology. Decis. Support Syst. 33(2) 2002: 111–126.
20 Keen PGW. Decision Support Systems: A Research Perspective. Center for Information Systems Research, Alfred P. Sloan School of Management: Cambridge, MA, 1980.
21 Sprague Jr. RH. A Framework for the Development of Decision Support Systems. Man. Inf. Sci. Quart. 4, 1980, 1–26.
22 Bhargava HK, Sridhar S, Herrick C. Beyond Spreadsheets: Tools for Building Decision Support Systems. Comput. IEEE 32(3) 1999: 31–39.
23 Eom SB, et al. A Survey of Decision Support System Applications (1988–1994). J. Oper. Res. Soc. 49, 1998: 109–120.
24 Jarrah AIZ, et al. A Decision Support Framework for Airline Flight Cancellations and Delays. Transp. Sci. 27(3) 1993: 266–280.
25 Abdelghany KF, Abdelghany AF, Ekollu G. An Integrated Decision Support Tool for Airlines Schedule Recovery During Irregular Operations. Eur. J. Oper. Res. 185(2) 2008: 825–848.
26 Simaria AS, et al. Allogeneic Cell Therapy Bioprocess Economics and Optimization: Single-Use Cell Expansion Technologies. Biotechnol. Bioeng. 111(1) 2014: 69–83.
27 Ungrin MD, et al. Rational Bioprocess Design for Human Pluripotent Stem Cell Expansion and Endoderm Differentiation Based on Cellular Dynamics. Biotechnol. Bioeng. 109(4) 2012: 853–866.
28 McCall MJ, Williams DJ. Developing Cell Therapies: Enabling Cost Prediction By Value Systems Modeling to Manage Developmental Risk. J. Commer. Biotechnol. 19(2) 2013; http://commercialbiotechnology.com/index.php/jcb/article/view/585.
29 Yang Y, Farid SS, Thornhill NF. Data Mining for Rapid Prediction of Facility Fit and Debottlenecking of Biomanufacturing Facilities. J. Biotechnol. 179, 10 June 2014: 17–25.
30 Lim AC, et al. A Decisional-Support Tool to Model the Impact of Regulatory Compliance Activities in the Biomanufacturing Industry. Comput. Chem. Eng. 28(5) 2004: 727–735.
31 Siganporia CC, et al. Capacity Planning for Batch and Perfusion Bioprocesses Across Multiple Biopharmaceutical Facilities. Biotechnol. Progr. 28, December 2013: 1–13.
32 Liu S, et al. Designing Cost-Effective Biopharmaceutical Facilities Using MixedInteger Optimization. Biotechnol. Progr. 29(6) 2013: 1472–1483.
33 Stonier A, et al. Decisional Tool to Assess Current and Future Process Robustness in an Antibody Purification Facility. Biotechnol. Progr. 28(4) 2012: 1019–1028.
34 Lim AC, et al. A Computer-Aided Approach to Compare the Production Economics of Fed-Batch and Perfusion Culture Under Uncertainty. Biotechnol. Bioeng. 93(4) 2006: 687–697.
35 Mustafa MA, et al. A Software Tool to Assist Business-Process Decision-Making in the Biopharmaceutical Industry. Biotechnol. Progr. 21(1) 2005: 320.
36 Lim AC, et al. Application of a Decision-Support Tool to Assess Pooling Strategies in Perfusion Culture Processes Under Uncertainty. Biotechnol. Progr. 21(4) 2005: 1231–1242.
37 Rouf SA, et al. Computer Simulation for Large-Scale Bioprocess Design. Biochem. Eng. J. 8(3) 2001: 229–234.
38 Farid SS, Washbrook J, TitchenerHooker NJ. Decision-Support Tool for Assessing Biomanufacturing Strategies Under Uncertainty: Stainless Steel Versus Disposable Equipment for Clinical Trial Material Preparation. Biotechnol. Progr. 21(2) 2005: 486–497.
39 Allmendinger R, Simaria AS, Farid SS. Multiobjective Evolutionary Optimization in Antibody Purification Process Design. Biochem. Eng. J. 91, 15 October 2014: 250–264.
40 Stonier A, et al. Integration of Stochastic Simulation with Multivariate Analysis: Short-Term Facility Fit Prediction. Biotechnol. Progr. 29(2) 2013: 368–377.
41 Suzanne S, Farid JW. Modelling Biopharmaceutical Manufacture: Design and Implementation of SIMBIOPHARMA. Comput. Amp. Chem. Eng. 31(9) 2007: 1141–1158.
42 Farid SS, et al. A Tool for Modeling Strategic Decisions in Cell Culture Manufacturing. Biotechnol. Progr. 16(5) 2000: 829–836.
43 Anuradha Rajapakse NJT-H. Integrated Approach to Improving the Value Potential of Biopharmaceutical R&D Portfolios While Mitigating Risk. J. Chem. Technol. Biotechnol. 81(10) 2006: 1705–1714.
44 George E, Titchener-Hooker NJ, Farid SS. A Multi-Criteria Decision-Making Framework for the Selection of Strategies for Acquiring Biopharmaceutical Manufacturing Capacity. Comput. Chem. Eng. 31(8) 2007: 889–901.
45 George ED, Farid SS. Strategic Biopharmaceutical Portfolio Development: An Analysis of Constraint-Induced Implications. Biotechnol. Progr. 24(3) 2008: 698–713.
46 Allmendinger R, Simaria AS, Farid SS. Efficient Discovery of Chromatography Equipment Sizing Strategies for Antibody Purification Processes Using Evolutionary Computing. Parallel Problem Solving from Nature: PPSN XII. Coello CAC, et al., Eds. Springer: Berlin–Heidelberg, Germany, 2012; 468–477; http://link.springer.com/ chapter/10.1007/978-3-642-32964-7_47.
47 Hourd P, et al. Manufacturing Models Permitting Roll-Out/Scale-Out of Clinically Led Autologous Cell Therapies: Regulatory and Scientific Challenges for Comparability. Cytother. 16(8) 2014: 1033–1047.
48 Feldman M, Maini RN. Anti-TNF Antibodies and Methotrexate in the Treatment of Arthritis and Crohn’s Disease. US6270766 B1, 7 August 2001; ww.google.com/patents/US6270766.
49 Feldman M, Maini RN. Anti-TNF Antibodies and Methotrexate in the Treatment of Autoimmune Disease. WO1998005357 A1 12 February 1998; https://www.google.com/ patents/WO1998005357A1?cl=de29 50 Ledford H. Blockbuster Drug Bows Out. Nature 480(7375) 2011: 16–17.
51 Brindley D, Reeve B, Mason C. Pharmaceutical Industry: Investors Unfazed By Drug-Patent Expiry. Nature 481(7381) 2012: 265.
52 Roberts M, et al. The Global Intellectual Property Landscape of Induced Pluripotent Stem Cell Technologies. Nat. Biotechnol. 32(8) 2014: 742–748.
53 Bravery C. A CATalyst for Change: Regulating Regenerative Medicines in Europe. The Delivery of Regenerative Medicines and Their Impact on Healthcare. Prescott C, Polak J, Eds. CRC Press: Boca Raton, FL, 2010; 285–312.
54 French A, et al. Enabling Consistency in Pluripotent Stem Cell-Derived Products for R&D and Clinical Applications Through Material Standards. Stem Cells Transl. Med. in press.
Rahul Rekhi contributed to this paper while a Marshall Scholar and graduate student in biomedical engineering at the University of Oxford and is now a staff economist at the Council of Economic Advisers (CEA) in the White House. (The CEA disclaims responsibility for any views expressed herein, and these views do not necessarily represent the views of the CEA or the United States.) Contributing equally was corresponding author James A. Smith, a research associate at the Oxford-UCL Centre for Advancement of Sustainable Innovation’s (CASMI’s) Translational Stem Cell Consortium (CSTCC) and a DPhil candidate at the University of Oxford’s Nuffield Department of Orthopedics, Rheumatology, and Musculoskeletal Sciences (NDORMS). Zeeshaan Arshad is an undergraduate student at St Andrews University. MacKenna Roberts is research associate at CASMI. Chas Bountra is professor of translational medicine in the Nuffield Department of Clinical Medicine and Associate Member of the Department of Pharmacology at the University of Oxford. Ian Bingham is founder of the IP Asset Partnership and a managing partner at IP Asset Ventures. Kim Bure is director of regenerative medicine at Sartorius Stedim Biotech and joint lead author with David A. Brindley, the Cooksey-Botnar-Saïd Fellow in Healthcare Translation at the University of Oxford’s NDORMS and the Saïd Business School; research fellow at the Harvard Stem Cell Institute; senior research fellow at the University College London Centre for Behavioral Medicine and member of the Stanford–UCSF FDA Centre for Regulatory Science and Innovation (CERSI).